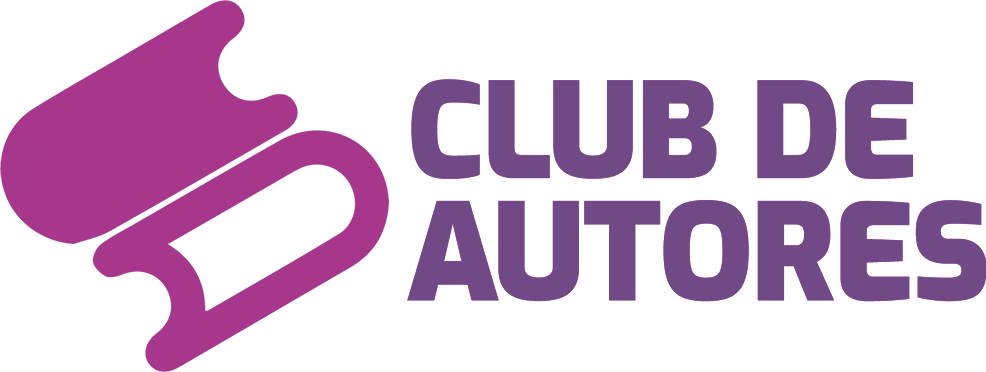
In Bayesian parameter inference, the goal is to analyze statistical models with the incorporation of prior knowledge of model parameters. The posterior distribution of the free parameters combines the likelihood function with the prior distribution using Bayes theorem. Usually, the best way to summarize the posterior distribution is to obtain samples from that distribution using Monte Carlo methods. Using these samples, you can estimate marginal posterior distributions and derived statistics such as the posterior mean, median, and standard deviation. HMC is a gradient-based Markov Chain Monte Carlo sampler that can be more efficient than standard samplers, especially for mediumdimensional and high-dimensional problems.
Gaussian process regression (GPR) models are nonparametric kernel-based probabilistic models. You can train a GPR model using the fitrgp function. Because a GPR model is probabilistic, it is possible to compute the prediction intervals using the trained model (see predict and resubPredict).
The general linear model (GLM) includes models of the analysis of variance and the simple and multiple covariance. That is, the GLM model includes the ANOVA, ANCOVA, MANOVA and MANCOVA models
Número de páginas | 277 |
Edición | 1 (2024) |
Formato | A4 (210x297) |
Acabado | Tapa blanda (sin solapas) |
Coloración | Blanco y negro |
Tipo de papel | Offset 80g |
Idioma | Español |
¿Tienes alguna queja sobre ese libro? Envía un correo electrónico a [email protected]
Haz el inicio de sesión deja tu comentario sobre el libro.